Frontiers of Science: Peter Norvig
Nov 13, 2024
Above: Peter Norvig. Credit: Todd Anderson
Using current AI large language models to teach the next generation of students
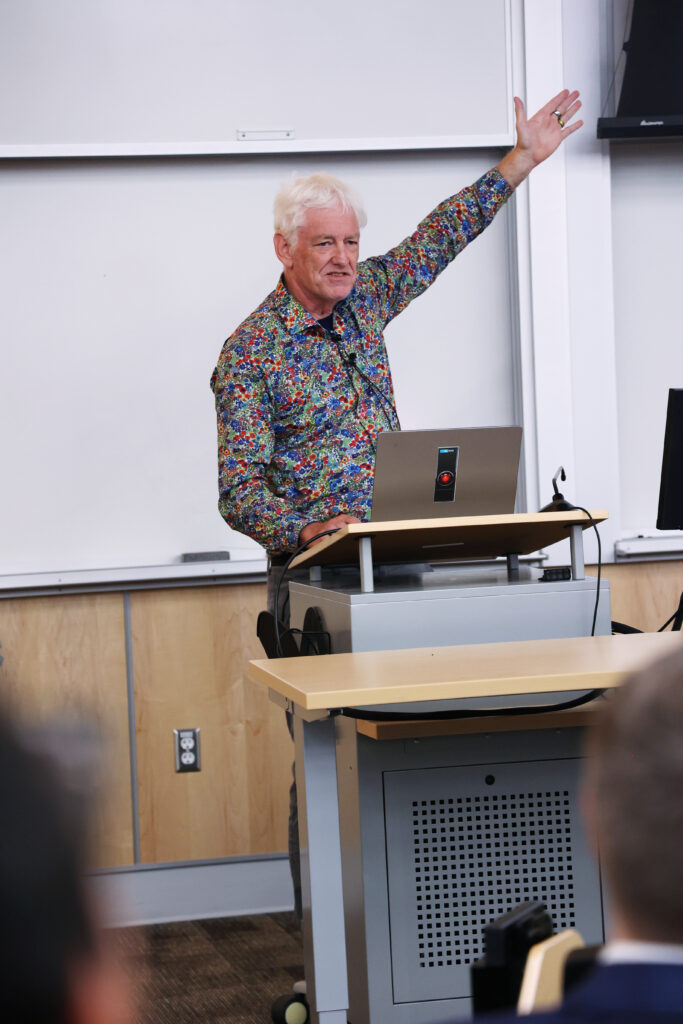
Peter Norvig. Credit: Todd Anderson
“I'm an AI hipster," said Peter Norvig who is known for wearing wildly patterned shirts borne of the Woodstock era. “I was doing it before it was cool, and now is our time.”
The featured speaker at the College of Science’s November 12 Frontiers of Science lecture series, Norvig was referring to the 2024 Nobel Prize in physics awarded to John Hopfield and Geoffrey Hinton for their pioneering work on neural networks, a core part of modern AI systems. Norvig’s address targeted how educators might use current AI large language models (LLMs) to teach the next generation of students.
To explore that question, Norvig, Distinguished Education Fellow at Stanford’s Human-Centered AI Institute as well as a researcher at Google, discussed the evolution of AI to an audience of 200. Norvig reflected back to 2011 when he and Sebastian Thrun pivoted from teaching a traditional AI course at Stanford to an online format where 100,000 worldwide enrolled. The free class featured YouTube videos and what’s called reinforcement learning, using machine learning that helped improve student performance by 10%.
In his lecture, Norvig cited Benjamin Bloom's "two sigma problem” in learning models and emphasized the importance of mastery learning “which means you keep learning something until you get it, rather than saying, 'Well, I got a D on the test, and then tomorrow we're going to start something twice as hard.'” Norvig also emphasized the importance of personalized tutoring.
“Really, the teacher’s role is to make a connection with the student,” Norvig said, “as much as it is to impart this information. That was a main thing we learned in teaching this class.”
These massive open online classes (MOOC) led to gathering massive data sets to help him and his colleague do a better job the next time. In “2024,” he said bringing us up-to-date, “we should be able to do more. And my motto now is we want to have an automated tutor for every learner and an automated teaching assistant for every teacher.”
But the objective for him is always the same: “I want the teachers to be more effective, to be able to do more, be able to connect more with the students, because that personal connection is what's important.”
Language, says Norvig, is humankind’s greatest technology, but “somehow we took this shortcut [in developing AI] of just saying, let's just [take] everything that mankind knows that's been written on the internet and dump it in. That's great. It does a lot of good stuff. There are other cases where we really want better quality, really want to differentiate what's the good stuff and what's not, and that's something we have to work on.”
Norvig acknowledges the challenge of obtaining necessary data to develop accurate student models. Unlike, for example, self-driving automobiles, which uses the data obtained through real-world-miles driven and repeating simulations of miles driven. He cited foundational work by the economist John Horton who is running experiments on computers using “agents” that duplicate a complex set of interactions between each other based on real-world experiments. “I think there's some kind of hope that we could do that kind of thing and have models of students that would tell us something,” he says. “We'd still have to verify that against the real world, but I think this would help a lot, because right now … we've [already] shown we can do 10% better” with student success averages.
There is no doubt that challenges will persist with improving and sufficiently complicating AI-generated content to be more helpful and humane when it comes to educating the next generation. In the context of LLMs, the “open world problem” refers to a scenario where the LLM needs to operate in an environment with incomplete or constantly changing information, requiring it to reason and make decisions without having all the necessary details upfront. It’s much like navigating a real-world situation with unknown variables and potential surprises.
The “open world problem” can’t be solved by traditional pre-programming of coders. There is something in between LLM’s “big empty box”—where you can ask anything you want, go in any direction— and top-down control of a MOOC where everyone ends up attempting to learn in the same way and doing the same thing. “We want the teacher to say, I'm going to guide you on this path, and we're going to get to a body of knowledge, but along the way, we're going to follow diversions that the students are interested in, and every student is going to be a little bit different.” Until the past two years, said Norvig, we never had any technology that could do that, and that “now maybe we do.”
Not only do we need to get AI right, Norvig continued, we need to ask, what does that mean? What is education? Who is it for? When do we do it? Where do we do it?
“The main idea is getting across this general … body of knowledge. But then there's also specific knowledge or skills. … Some of it is about reasoning and judgment that's independent of the knowledge. Some of it is about just getting people motivated … Some of it is about civic and social coherence, being together with other people and working together, mixing our society together.”
It’s a tall order for AI engineers, teachers and students.
For Norvig, the long game is underwritten by the importance of understanding long-term educational goals and balancing AI's benefits with human connections. It’s nothing short of redefining what an education means.
In the 80s, he says, it was about algorithms telling us things; in the “oughts” it was about the showing of big data; and now in the 20s it has turned to the philosophical: What do we need and what do we want in our real and AI world to prepare students for the future and, once they enter the workforce, to distinguish tasks and jobs. (Changing the mix of tasks, he says, will undoubtedly continue.) What technology do we want to invest in and how will it impact employment?
In his presentation, Norvig engagingly careened from big scale to micro-scale almost in the same sentence, but it’s what the sector is being asked to do at this inflection point in AI technology: mixing the technological with the philosophical, asking hard questions, and thinking inside and without that “open box.”
Fortunately, in the good professor/director of “human-centered AI,” we have a guide and a cheerleader. Not only are his wildly printed shirts easy on the eye, but, the audience was told in the evening’s introduction that he founded the ultimate frisbee club at Berkeley when he was a graduate student.
For Peter Norvig, the self-described “AI hipster,” he’s clearly known for a long while what was cool, “before it was cool.”
Frontiers of Science is the longest continuously running lecture series at the University of Utah, established in 1967 by U alumnus and physics professor Peter Gibbs.
by David Pace